MLflow
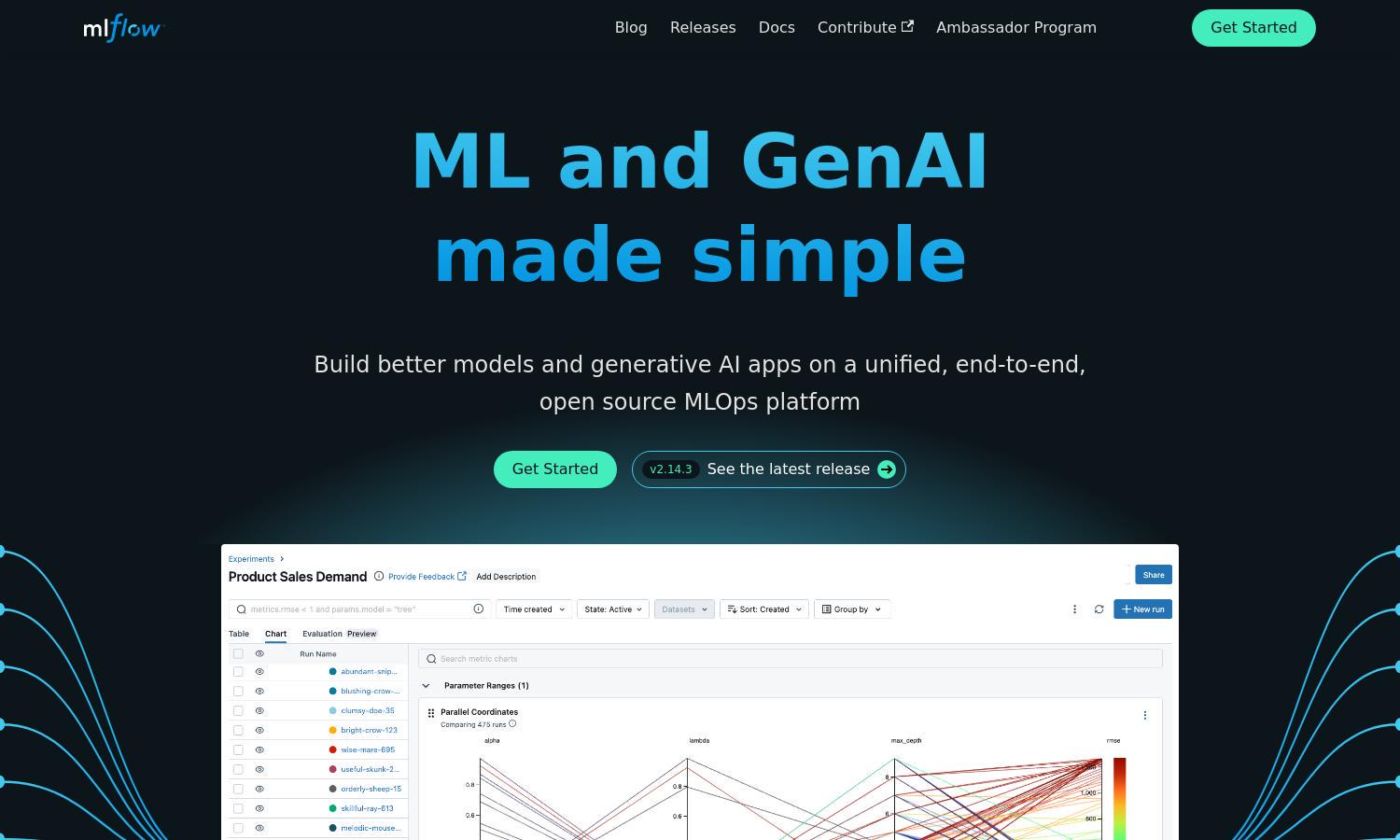
About MLflow
MLflow is an open-source MLOps platform designed for data scientists and ML engineers. It facilitates the management and deployment of machine learning and generative AI projects through experiment tracking, model registry, and observability features. Users benefit from a streamlined workflow, enhancing productivity and collaboration.
MLflow offers a free tier and additional paid plans with advanced features. Standard access provides core functionalities, while premium options unlock enhanced collaboration tools and priority support. Upgrading allows users to maximize their ML workflow capabilities, ensuring they have the resources needed for efficient model management.
MLflow's user interface is designed for ease of navigation, with a clean layout that enhances the browsing experience. Key features like dashboards for experiment tracking and intuitive model registration processes enable users to efficiently manage their ML projects, making it accessible for all skill levels.
How MLflow works
Users start by signing up for MLflow and exploring the user-friendly interface. They can create projects, track experiments using intuitive dashboards, and manage models through the model registry. The platform also provides deployment options, enabling seamless collaboration among team members throughout the machine learning lifecycle.
Key Features for MLflow
Unified MLOps Platform
MLflow serves as a unified MLOps platform, bringing together machine learning and generative AI functionalities. Users can effortlessly manage experiments, track progress, and deploy models, ensuring a consistent workflow. This unique capability enhances collaboration and efficiency, solidifying MLflow's position as a leader in MLOps.
Experiment Tracking
MLflow offers robust experiment tracking functionalities, allowing users to log parameters, metrics, and artifacts seamlessly. By capturing detailed experiment data, MLflow empowers data scientists to analyze results effectively, iterate on model designs, and make informed decisions, enhancing the overall success of machine learning projects.
Model Registry
The Model Registry feature in MLflow facilitates organized model management, enabling users to store, version, and share models with ease. This feature allows teams to maintain clarity across different versions, ensuring that only the best-performing models are deployed, ultimately improving workflow efficiency and collaboration.